News — A toy tool belt fastened around his small waist, Michael Scott Fischer stood in front of his southern Miami-Dade County home one day in the summer of 1993, ready to lend a hand to the workers who were repairing his family’s storm-damaged house.
It had been nearly a year since Hurricane Andrew destroyed a large portion of it, forcing him and his parents to live elsewhere until repairs could be made.
“Some of my earliest memories were of seeing people totally remodeling our home and believing I could use my childhood toolkit to help them,” Fischer said. “I had a natural curiosity, wondering how something could be so powerful and destructive that we couldn’t live in our home for so long.”
Fischer’s toy drill, hammer, and screwdriver couldn’t make a difference back then. But today, as a researcher at the , he is employing a much more powerful tool to determine why some hurricanes rapidly intensify: artificial intelligence.
As part of his strategy, he is enhancing current storm data by utilizing historical measurements of past hurricanes. Those measurements include wind speeds, temperature, humidity, and other atmospheric readings gathered by buoys, satellites, and hurricane hunter aircraft over the past several decades.
“I use a variety of observational data sets and data science methods to identify commonalities among subsets of storms that have undergone rapid intensification,” explained , an assistant professor in the Rosenstiel School’s Department of Atmospheric Sciences. “And hopefully, with that knowledge, we can provide tools that would improve our prediction of tropical cyclones.”
Fischer’s use of AI extends beyond the study of hurricane rapid intensification. In a study funded by the National Oceanic and Atmospheric Administration (NOAA), he is trying to improve the quality of radar observations collected by hurricane hunter aircraft. “Those planes accumulate a wealth of radar readings, but some of those observations are not necessarily meteorological data. Some of it could be noise from the ocean surface or artifacts of the radar system. So we’re developing a machine learning technique to quality control that data and classify whether it’s meteorological or non-meteorological in nature,” said Fischer, who is the principal investigator on the project and is collaborating with scientists from Colorado State University.
Initial results are promising, with the method surpassing the capabilities of humans. “It would take a human multiple hours to inspect the radar data that the machine learning program can quality control in just a few minutes,” Fischer said.
In a second study for which he is seeking funding, Fischer is training computers to analyze decades worth of satellite data from storms, with the goal of building a 3D structure of an active hurricane in the Atlantic basin without having aircraft fly into the system.
“Airborne reconnaissance over the Atlantic is routine once a storm is located west enough that we can get within range of aircraft. But even then, we don’t always have planes out there. The hurricane hunters can spend hours flying within a storm, taking readings. But eventually, they must return to base, refuel, and deploy a new crew. And that takes time,” Fischer said. “Our method would allow us to always have a full three-dimensional structure of what a storm looks like. And it would be particularly helpful not only in the Atlantic basin but also in other basins worldwide where hurricanes form but where there are not always routine aircraft observations.”
Fischer is part of the rapidly developing field in which AI models are being used to forecast weather. From hurricanes and heatwaves to rainfall and drought, those models are predicting in minutes what used to take hours. “With AI, my ultimate goal is to save lives and safeguard infrastructure by improving the advanced warning times for populations in the path of storms,” Fischer said.
He holds a joint appointment in the University’s , combining his work at the Rosenstiel School with the computing power of that Coral Gables Campus-based center to tackle the problem of why tropical cyclones rapidly intensify.
, a senior research associate at the Rosenstiel School’s and with the Hurricane Research Division of NOAA’s Atlantic Oceanographic and Meteorological Laboratory, is also part of that revolution, using AI techniques to enhance hurricane forecasts and to study intensity changes in storms.
“I became fascinated by weather while growing up in Taiwan and experiencing how typhoons impacted my homeland,” said Ko, who holds advanced degrees in electrical and computer engineering and in meteorology from the University.
Still, there are challenges involved in applying AI technology to storm forecasting. “The hard part about using AI models is training them on past historical data,” Fischer said. “Extremely powerful supercomputers are used to train the models, and once they are trained, they can operate rather quickly. It’s akin to stored memory, and then it calls on that memory to build a forecast. And the AI models can run much quicker than traditional numerical weather prediction models and could actually be run on a laptop.”
The models are proving to be remarkably accurate. Google DeepMind’s AI model, GraphCast, for example, correctly predicted that Beryl—the first hurricane of the 2024 Atlantic hurricane season and the earliest Category 5 cyclone ever recorded in the Atlantic—would make landfall in Texas when a top European model warned that a landfall in Mexico was likely.
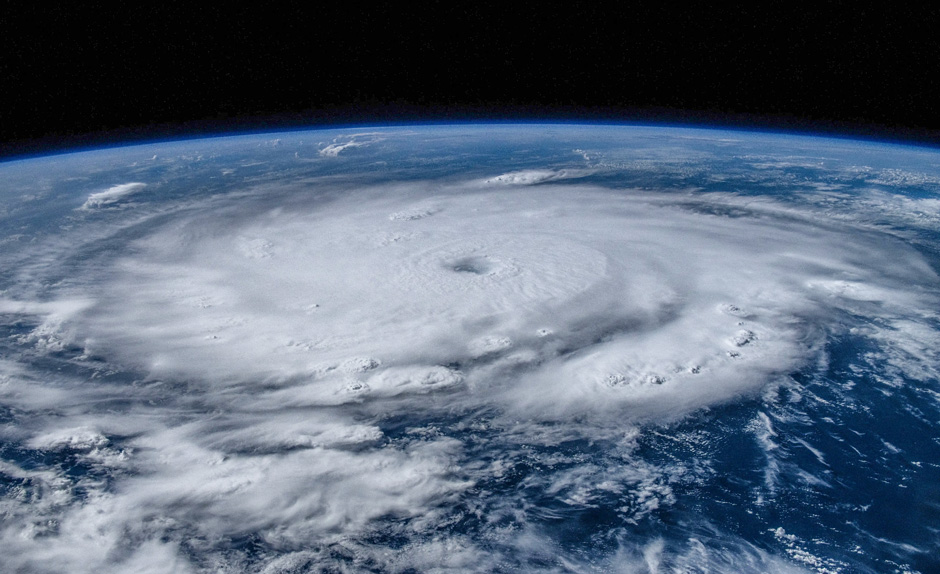
Hurricane Beryl on July 1, 2024, as captured by astronauts aboard the International Space Station. An AI model correctly predict the storm's U.S. landfall. Photo: NASA
Other hurricane forecasts generated by AI models have been compared to forecasts made by traditional numerical weather prediction models, with the former producing more accurate outlooks. The AI model Pangu-Weather produced smaller forecast track errors for a group of hurricanes at all lead times through five days when compared to the European Centre for Medium-Range Weather Forecasts model, Fischer pointed out.
AI also has the potential to benefit poorer countries that might not have the resources to operate their own local or global weather forecasts. “But that requires the output from AI models to be publicly available for either free or at a very cheap price,” Fischer said. “And an additional complicating factor is how those forecasts are being communicated to those in poorer regions of the world. We could have models that produce more accurate forecasts, but if people such as farmers in Global South don’t receive word of those forecasts, they won’t benefit from them. So public dissemination and communication of these forecasts is critical.”
But as remarkably fast and brainy as AI is proving to be in forecasting hurricanes and other weather events, it will never replace humans, Fischer said.
“It’s just another tool in our toolkit—potentially, maybe our strongest tool as we continue to refine it,” he explained. “Where humans will continue to be needed is in conveying information about an approaching cyclone or other severe weather threat to the public in a way the people can understand it. AI could have the perfect forecast of what’s going to happen, but if that forecast is not easily interpretable, humans will still be needed to convey the threat to residents in the path of a storm. What kinds of precautions should they take to prepare for the storm? Should they evacuate, and where are the nearest shelters located? That’s where we’re needed. And I don’t see humans being replaced on that front any time soon.”